This intuitive AI can sort through scans to diagnose stroke, brain haemorrhage, brain tumors and skull fractures.
A Tsinghua team has developed a self-taught deep learning system that can detect four different conditions – stroke, brain haemorrhage, brain tumors and skull fractures. Their artificial intelligence (AI) performed at 96% accuracy, the same rate as radiologists1.
Tsinghua's Institute for Brain and Cognitive Sciences have helped create an AI that uses less man power to learn to detect a number of conditions linked to the head using computed tomography images. These conditions include stroke, brain haemorrhage (pictured), brain tumors and skull fractures.
Qionghai Dai, a professor at Tsinghua’s Department of Automation, explained that the AI learned to read head scans without a great deal of guidance from radiologists.
Growing demand
Stroke, brain haemorrhage, brain tumors and skull fractures alter the structure of the head and brain, increasing the risk of permanent brain damage or death, explains Dai. Since it was developed in the 1970s, computed tomography (CT) scanning has used X-rays to produce multiple cross-sectional images of the brain to assess these types of abnormalities. Hundreds of millions of CT scans are performed every year, putting increasing pressure on doctors and radiologists. “Analysing all these images is challenging and labour-intensive,” says Dai, “and many sites lack experienced radiologists to do this extra work.”
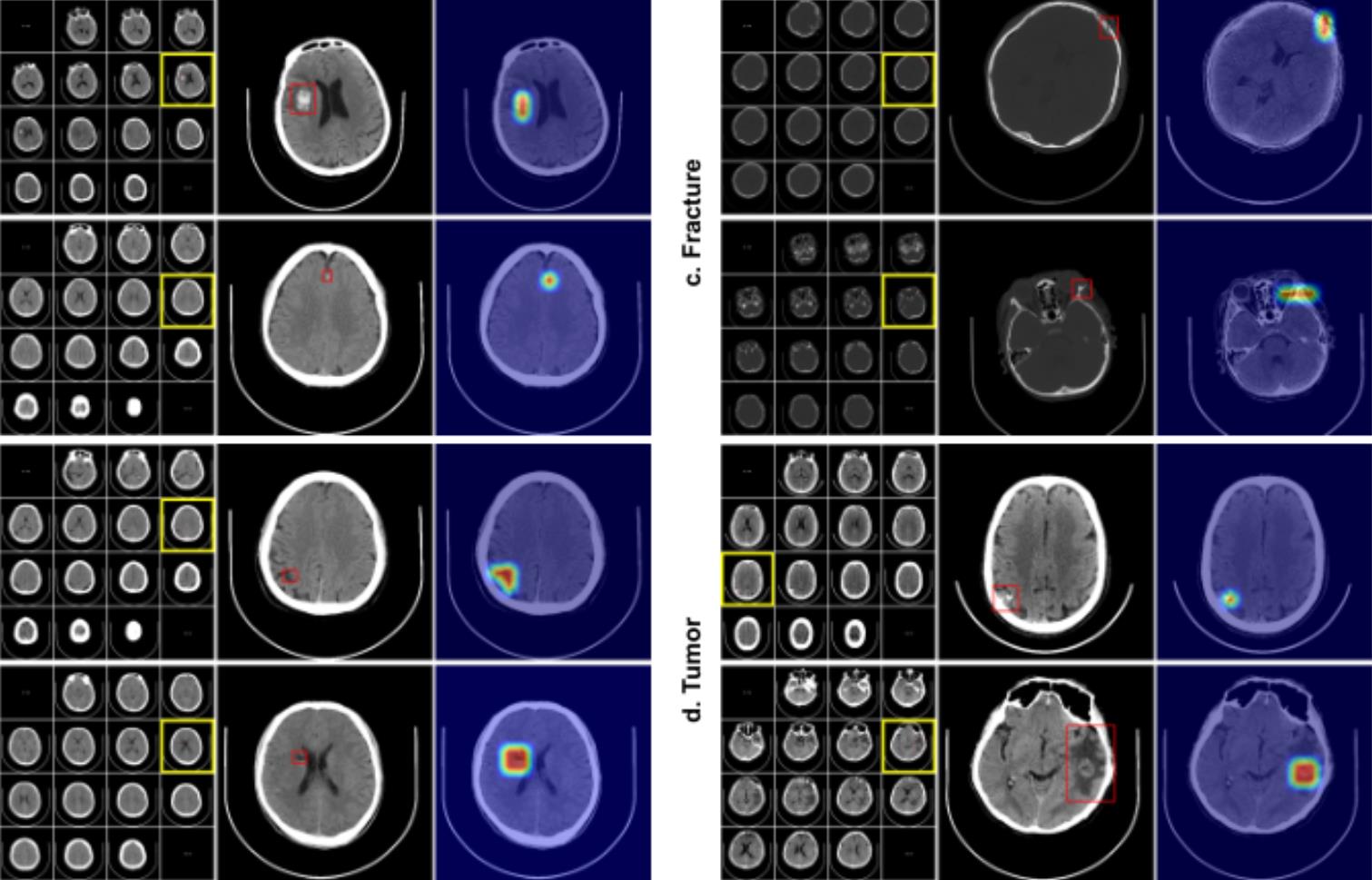
Artificial intelligence researchers have been testing whether machines could be taught to detect disorders related to the head. Supervised learning demands vast amounts of annotated training data, in which specialists have already labelled the affected areas, says Dai. “This can be very expensive, so most studies can only build small datasets. This results in AIs that can only diagnose one or two specific diseases, and only perform well within the walls of one medical centre,” he says.
In recent years, a few studies have achieved the gold standard of above 95% diagnostic accuracy, but only for specific disorders, such as brain haemorrhages, he adds. These AIs were trained on between 1,000 and 4,000 CT scans that had all been heavily annotated by radiologists.
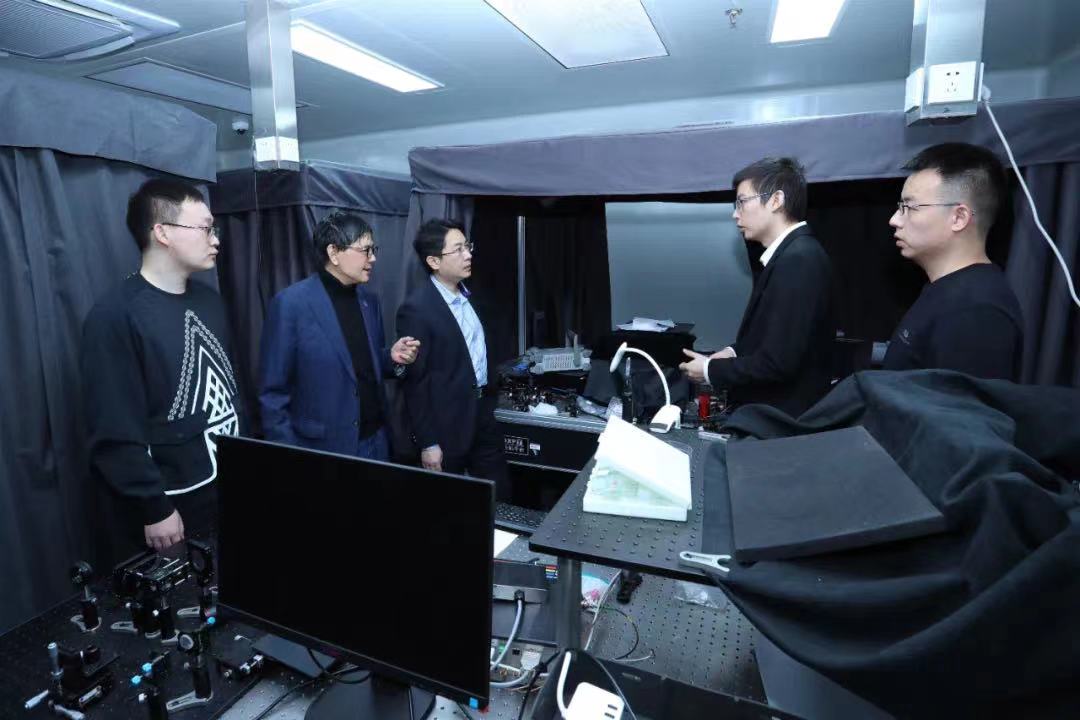
However, it is possible to build considerably larger training datasets without any expert guidance by using weak annotation, whereby messy or imprecise information, such as written descriptions, are used to generate labels, says Dai.
It was using this technique that he and his team developed their annotation-free deep learning system.
Hungry AIs
But this type of deep learning is extremely data-hungry, using multiple layers of computations that allow the model to learn by itself. To develop their system, the researchers collected more than 100,000 CT scans, along with their relevant diagnostic reports.
The scans, which sometimes revealed multiple disorders, included roughly 20,000 haemorrhages, 25,000 strokes, 19,000 fractures, 3,000 tumours, and 45,000 normal cases.
The team used automatic keyword matching on the reports to generate labels for the scans, which they fed into their deep learning algorithm, RoLo (Robust Learning and Localized Lesion). This is called ‘weakly supervised’ machine learning, as it draws on disorganised and imprecise information to create training data. “Weak annotation can lead to wrong labels in training data and working at the scan-level labels means it does not show the specific location of relevant head lesions,” says Dai. “But it is much easier to create a large-scale training set this way, so we can shift the cost from expert labelling to novel AI algorithm design,” he says. Bigger data sets and better algorithms will help reduce the influence of errors, he adds.
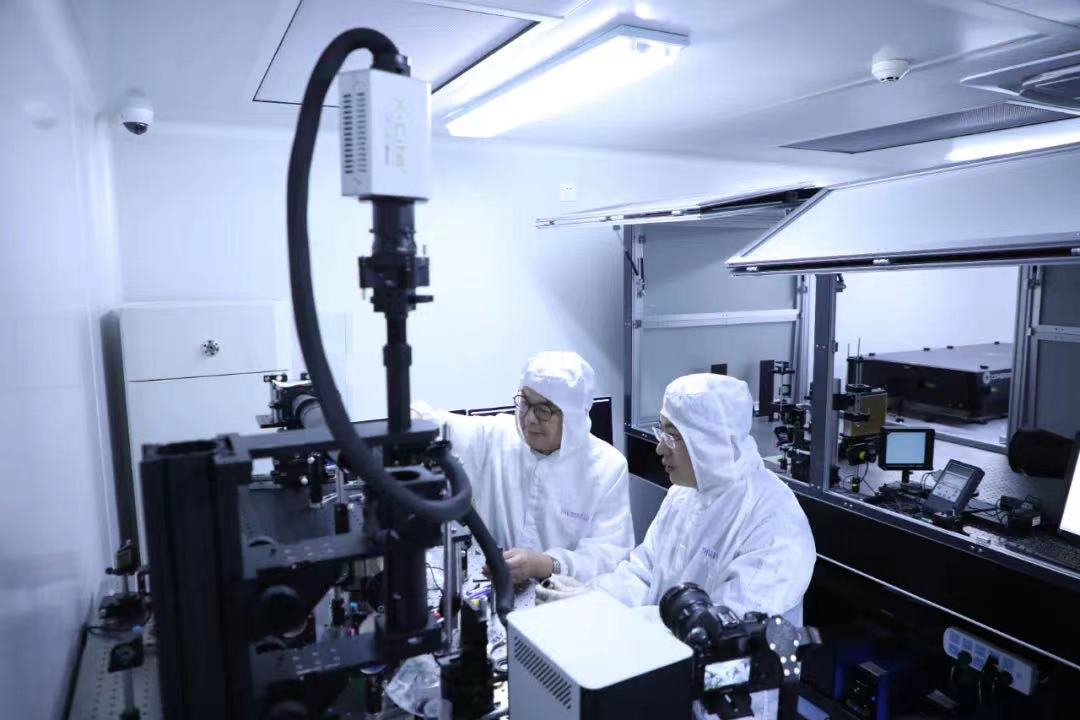
To check whether their AI worked in a range of settings, Dai’s team tested it on about 3,000 CT scans taken from one hospital in China; 1,500 from several different hospitals; 1,500 from different scanning set-ups; and 500 from a dataset from India. They found their AI could correctly diagnose and distinguish between the four head disorders equally well across the different datasets. It averaged more than 96% accuracy, matching the performance of four radiologists who assessed the scans by eye.
Having tested RoLo extensively in the lab, Dai and his team are keen to iron out the logistics and legalities of bringing their new AI into hospitals. “We want to make an affordable and accurate AI system that can be used routinely in clinics and hospitals around the world to help research and diagnose head disorders,” says Dai. “Now we know that we can build an AI for diagnosing four different disorders, we are keen to develop new AI theories, design state-of-the-art AI algorithms, and build a practical AI system that can detect even more conditions.”
Reference
Guo, Y., He, Y., Lyu, J., Zhou, Z., Yang, D. et al. Deep learning with weak annotation from diagnosis reports for detection of multiple head disorders: a prospective, multicentre study Lancet Digital Health 2022 4(8), E584-E593 (2022) doi: 10.1016/S2589-7500(22)00090-5
Editor:Guo Lili